Pacing the commitment of private asset investments is notoriously challenging compared to other asset types. Net growth expectations are impacted by the cycle of remaining capital left to call and outflows via distributions, not to mention they take years to fully realize. With that in mind, FRG expanded our base private cash flow forecasting tool, PCF, to include a flexible yet robust pacing optimizer.
In my last blog post, FRG’s Next-Gen Private Capital Forecasting Model vs the Standard Yale’s Takahashi-Alexander, I outlined how FRG’s PCF model improves upon the industry standard Takahashi-Alexander (aka Yale) model. In a nutshell, it accomplishes this by incorporating not only fund characteristics, but also historical trends and macroeconomic scenarios. The resulting models are then applied to a Monte Carlo simulation to obtain distribution statistics for future cash flows and performance metrics.
FRG’s pacing optimization tool combines our base forecast with a client’s allocation targets and desired investment strategy to determine how much they will need to commit in the future to keep their allocation in line. Required inputs to our pacing tool include the target allocations along with anticipated future fund vintages and classifications.
Let’s walk through a brief example. The chart below shows the private allocation through time for a hypothetical client. The dotted lines represent the client’s allocation targets, while the solid lines represent the forecasted allocation values. As expected, the Net Asset Value (NAV) drops as funds fully mature, resulting in diminishing allocation across the board.
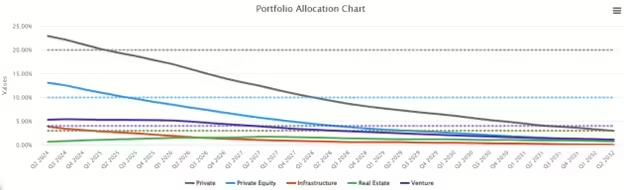
Wanting to maintain their allocation targets, this client plans to commit to several new funds over the next few years, but they aren’t sure how to optimize their budget. In comes FRG’s pacing optimization tool. All they need to do is specify which sub-asset classes and years they want to commit to, and the tool will not only provide the future commitments needed to keep their allocation targets afloat, but also automatically generate an updated forecast with those commitments assigned. Now, if this client checks their updated allocation chart, it will look something like this:
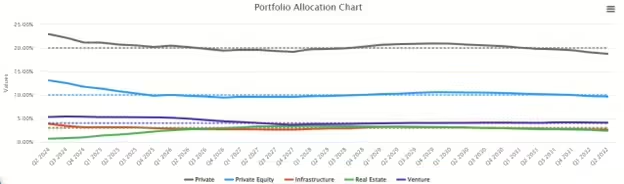
The results include details on exactly what, when and where commitments are added to achieve these results. And just like that, this client has the solution to one of the more challenging aspects of private asset management.
FRG’s pacing optimizer is a powerful, easy-to-use tool, but what really makes it stand out is its customizability. Weights for vintage years can be specified to adjust the pace of commitments, and trading rules and rebalance timing can be set. What’s more, allocation can be viewed at the total portfolio level, including other asset classes outside of private.
Interested in learning more? Request a demo or sign up on our Resources page to access our white paper, Optimal Pacing for Private Capital Portfolios.
Jenny Harrod is a business analytics consultant at FRG. Since joining FRG, she has become involved with FRG’s Private Capital Forecasting solution, assisting with implementation for clients and providing ad-hoc analysis. She also performs exploratory data analysis, builds machine learning algorithms, and writes and maintains technical documentation.