This blog series explores the stages of the Model Development Lifecycle so that you understand the components of model development and maintenance as well as where potential sources of model risk can reside. Read the first post here for an introduction to the series and why we are likening this process to the creation of a cookie recipe.
Overview
In this blog about the MDLC the focus is on: Data Assessment.
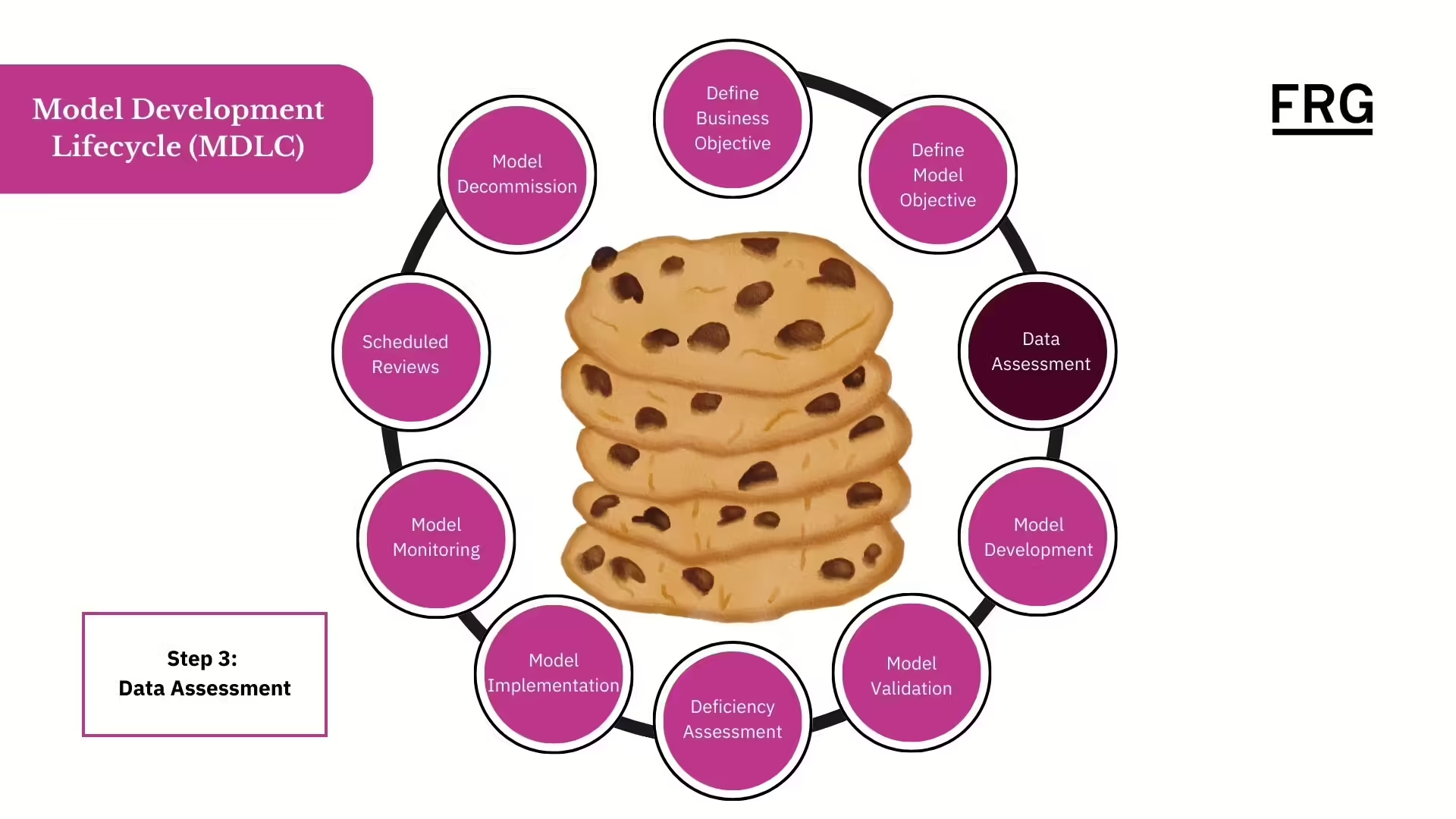
Cookie’s Story: A little bit of this and a little bit of that
Cookie walked into her test kitchen and immediately felt herself relax. This was her place. Stainless steel counters, sinks, and appliances glistened in the kitchen’s light. On the walls hung the tools of a baker—measuring cups, sifters, beaters, whisks, rolling pins of varied sizes, cookie cutters, wooden spoons, and spatulas. And the aroma…so much had been baked in this kitchen that it always smelled like cookies.
There were several industrial refrigerators, a freezer, and a door leading to a pantry. She grabbed a cart and headed through the door, flipped on the light, and smiled. While baking was a joy—the creation of something wonderful—she used most of her creativity in selecting ingredients. Granted, a cookie needed the basics: flour, sugar, and some type of fat. But what makes the cookie interesting? What puts the “mm” in “yummy”? It was the non-basic ingredients.
Cookie started placing ingredients on her cart. She grabbed dark, semi-sweet, and milk chocolate chips. She reached for pecans but then decided to forego those with this bake. The recipe needed to be as simple as possible. She added granulated sugar, light brown sugar, and turbinado sugar. The latter was added because she was curious how it might work with other ingredients. On her way out of the pantry she grabbed vanilla, and almond and lemon extracts.
She stopped at a refrigerator to gather eggs, milk, butter, and Irish butter then rolled her cart to the side of the central baking table. She unloaded the cart, gathered her baking tools, and then put on a baker’s cap. The baker’s cap, a gift from her parents when she was just starting the business, had seen many years. It was worn, no longer a bright white, but was her good luck charm. Every time she created a new recipe, she wore it.
Cookie cracked her knuckles and rotated her shoulders. The selection of ingredients was the crucial step of the recipe process. Many people assumed the crucial step was the fine-tuning of the amounts of ingredients. In reality, it was the mix. Getting the correct combination of flavors was essential. If she didn’t get that right the recipe wouldn’t be needed because no one would buy the cookies.
Cookie rolled her shoulders once more then started combining the ingredients to find which ones worked best together.
Relating it to the MDLC
The third stage of the MDLC is about understanding the data.
There are two main actions at this stage. The first is to process the data. This involves sourcing, reviewing, cleaning, and transforming the data. The goal is to prepare the data.
Why is data preparation important for model development?
Preparation of data ensures data used in modeling is of the best quality (e.g., variables contain the correct values, data comes from reliable sources) for the development work at hand.
The second is to analyze the data (i.e., exploratory data analysis, EDA). This involves identifying:
- correlations between variables
- the presence of anomalies
- patterns that might support the model build process.
The goal is to understand the information in the data.
Why is data understanding important for model development?
Understanding the data allows model developers to determine if the data available is suitable for model development (e.g., aligns with business intuition, captures relevant behaviors).
For Cookie, it was all about the ingredients (i.e., her data):
- She gathered common and uncommon ingredients (i.e., sourcing and reviewing) because she wanted to make sure she found the best ones to satisfy her recipe objective.
- She combined them (i.e., EDA) to understand how they interacted.
What happens next in Cookie’s kitchen? Our next blog will cover how the model development process builds upon the data assessment that Cookie did with her ingredient testing.
Jonathan Leonardelli, FRM, Director of Business Analytics for FRG, leads the group responsible for business analytics, statistical modeling and machine learning development, documentation, and training. He has more than 20 years’ experience in the area of financial risk.
RELATED:
What is the Model Development Lifecycle, or, What’s Baking at FRG?
The Model Development Lifecycle (MDLC): Defining the Business and Model Objectives